Objectives: To demonstrate the concept of filtering in the frequency domain and how this affect the quality of images.
Tools: Scilab with SIP toolbox
Procedure: We begin by finding the FTs of pairs of apertures such as the ones we have below.
As with the previous activity, the FTs of the various patterns appear as a convolution of the paterns of a single aperture. It is also of note that the Gaussian FT is still a Gaussian but is tranformed in the frequency space. That is, a wider, more spread out Gaussian function has a narrower transform in the FT space.
The next set of examples illustrate the us of filtering in the Fourier space to cancel out certain undesirable components in the images.For example, the image of the moon below has a set of vertical lines which are very pronounced. Also it has some horizontal scan lines which may be an artifact of the mosaic process. In order to remove these, we implement the following steps:
1. Take the FT of the image.
2. Create a mask to remove certain components.
3. Shift (fftshift) the mask so that the lower order componenents are on the outer portions of the image.
4. Multiply the mask and the FT
5. Take the FT of the product. and normalize.
For this image we use a cross shaped filter to cancel out the periodic, cosinusoidal frequencies in the x and y direction. Note that the mask does not cover the DC component as this contains a lot of information about all the points on the object. Hence if it is removed we lose valuable information. The resulting filtered image is shown below the original. Note that the lines have been removed and the image is cleare. However since we inadvertently removed other useful components, the image has less contrast and detail in some parts than the original.
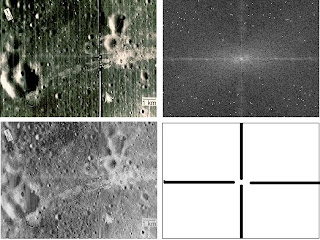
On the other hand, we a decidedly more difficult image with the next one which is a patch from a painting on stretched canvas. The task is to remove the weave pattern to enhance the actual image. For this we work directly with the FT of the image. The bright spots symmetric about either the x or y axis are the frequencies we want to dscard for these indicate periodic, cosinusoidal patterns in image space. Thus we place circular masks at the positions of these points. We see that when such a mask is used to filter the image, we get a weave free image. Also if we now take our mask and use the centers as the locations of bight spots, that is if we invert the mask, then take the FT, we come up with a weave pattern similar to what we removed from the original image.

For the last section, we attempt to enhance the ridges and lines on an image of a fingerprint. From the FT we discern that our region of interest is the band like structure centered about the DC component. We make a mask to leave only this and the DC component. We see that indeed we can recover the medium frequency components and enace the lines while removing most of the high order noise.
Evaluation: Since all the images were adequately enhanced using FT methods, a grade of 10 is applicable.
Acknowledgements: I would like to thank Misters. Garcia, Gubatan, Panganiban and Cabello for their helpful inputs.
Rating: Since all the results tally with what is expected and the images are well filtered, a score of 10 is warranted.